In today’s data-driven world, organizations are generating and collecting vast amounts of data at an unprecedented rate. From customer interactions to operational metrics, data is the backbone of decision-making processes. However, the value of this data is contingent upon its quality. Poor data quality can lead to misguided strategies, lost revenue, and damaged reputations. In this article, we will explore why data quality matters and how to avoid the pitfalls associated with poor data practices.
Understanding Data Quality
Data quality refers to the condition of a dataset, evaluated based on various factors such as accuracy, completeness, consistency, reliability, and timeliness. High-quality data is accurate, up-to-date, and relevant to the intended purpose. Conversely, low-quality data can lead to erroneous conclusions and ineffective actions.
Key Dimensions of Data Quality:
- Accuracy: Data must accurately represent the real-world scenario it intends to depict. For example, a customer’s address must be correct to ensure successful delivery of products.
- Completeness: A dataset is considered complete when all necessary information is present. Missing data can lead to skewed analyses and poor decision-making.
- Consistency: Data should be consistent across different datasets and systems. For instance, a customer’s name should appear the same in all records, regardless of the database.
- Reliability: Data should come from a trustworthy source. Data collected from unreliable sources can introduce errors and biases.
- Timeliness: Data must be up-to-date to be relevant. Outdated information can mislead decision-makers, especially in fast-paced industries.
The Consequences of Poor Data Quality
The implications of poor data quality can be severe and multifaceted. Here are some common pitfalls organizations face:
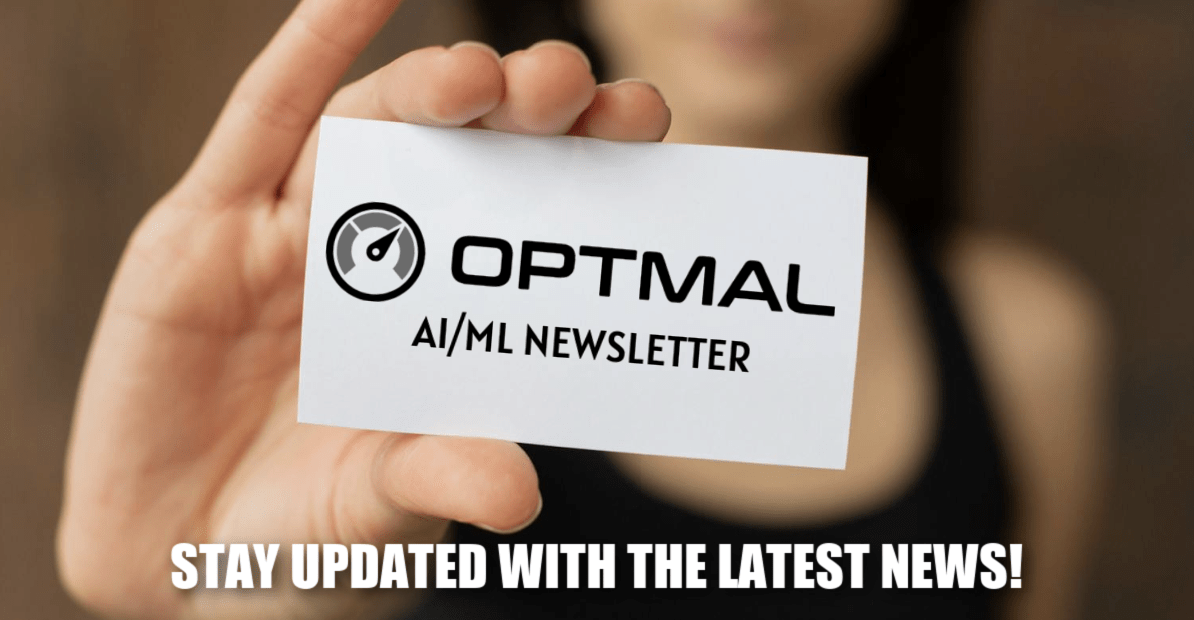
- Informed Decision-Making: Decisions based on inaccurate or incomplete data can lead to misguided strategies. For example, a retail company relying on outdated sales data may misjudge inventory needs, resulting in stockouts or overstock situations.
- Financial Loss: Poor data quality can directly impact an organization’s bottom line. A study by IBM estimated that poor data quality costs the U.S. economy around $3.1 trillion annually. Companies may incur expenses related to fixing data errors, lost sales opportunities, and compliance fines.
- Damaged Reputation: In the age of social media, news spreads quickly. If a company is found to be using inaccurate data in its marketing or reporting, it can lead to a loss of customer trust and brand loyalty.
- Regulatory Compliance Issues: Many industries are subject to strict regulations regarding data management. Poor data quality can lead to non-compliance, resulting in legal repercussions and hefty fines.
- Inefficiencies: Low-quality data can create inefficiencies in operations. Employees may spend excessive time correcting errors or searching for missing information, diverting resources from more productive tasks.
Strategies for Ensuring Data Quality
To avoid the pitfalls associated with poor data quality, organizations must adopt proactive strategies:
- Establish Data Governance: Implementing a data governance framework helps ensure accountability and ownership of data quality across the organization. This includes defining roles and responsibilities for data management.
- Regular Data Audits: Conducting regular audits of data can help identify inaccuracies, inconsistencies, and gaps. This process should include checks for data accuracy, completeness, and timeliness.
- Invest in Data Quality Tools: Utilizing data quality tools can automate the process of data cleansing, validation, and enrichment. These tools can help identify duplicates, standardize formats, and fill in missing information.
- Train Employees: Educating employees about the importance of data quality and best practices for data entry can significantly reduce errors. This training should be ongoing, as data management practices evolve.
- Encourage a Data-Driven Culture: Fostering a culture that values data quality can lead to better practices throughout the organization. Encourage teams to prioritize data accuracy and share insights on data quality improvements.
Conclusion
In a world where data drives decisions, the importance of data quality cannot be overstated. Organizations must recognize the potential pitfalls of poor data practices and take proactive steps to ensure their data is accurate, complete, consistent, reliable, and timely. By investing in data quality initiatives, companies can make informed decisions, enhance operational efficiency, and ultimately drive better business outcomes. Remember, high-quality data is not just a nice-to-have; it’s a necessity for success in today’s competitive landscape.
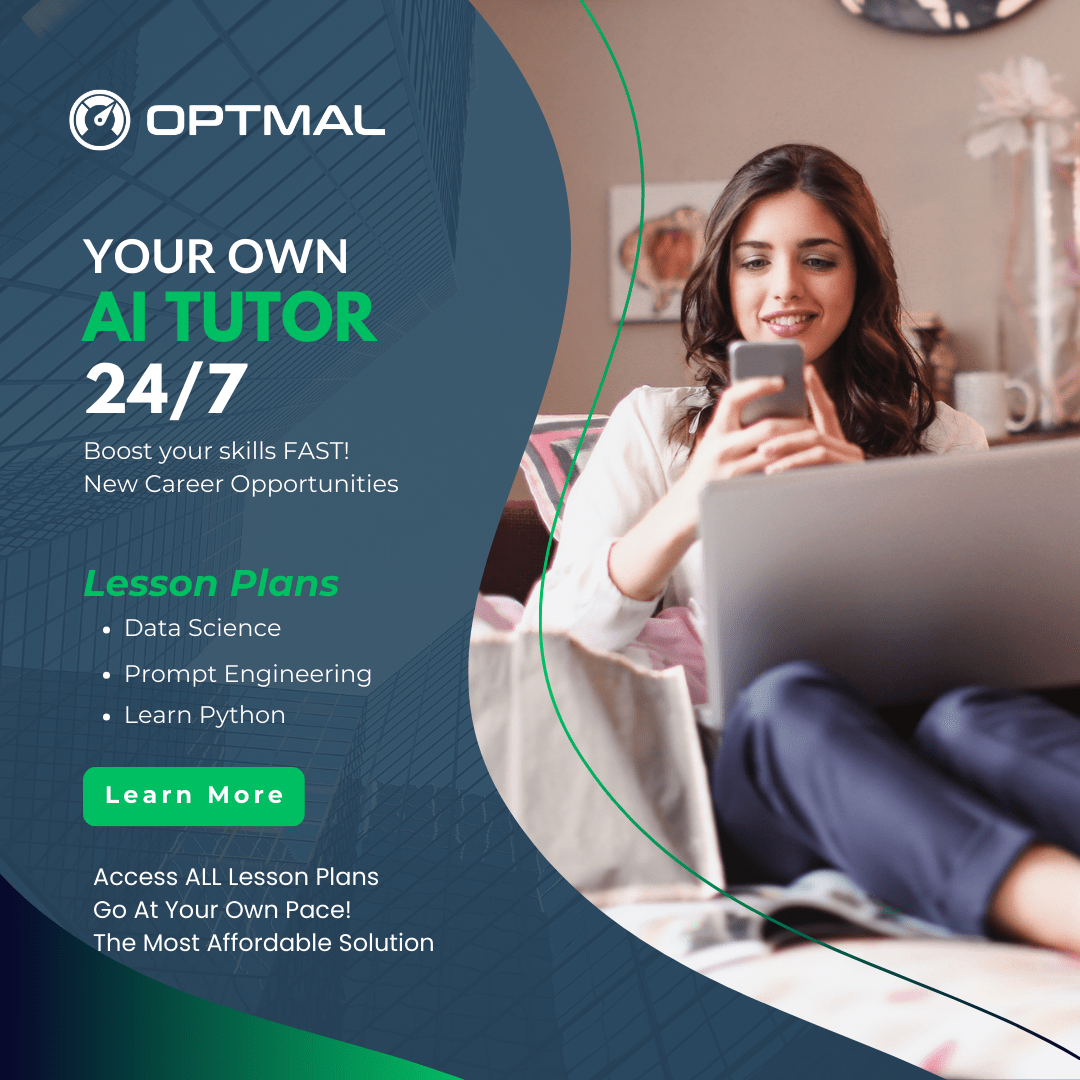
Boost Your Skills in AI/ML